Spatial distribution of local patch extinctions drives recovery dynamics in metacommunities
Texte intégral
Figure
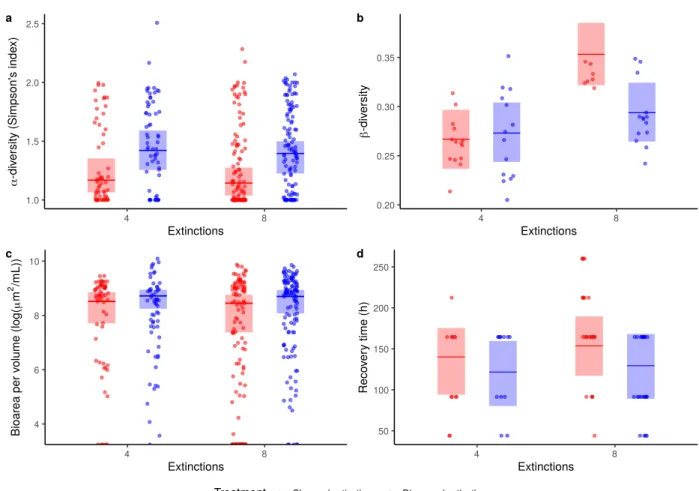



Documents relatifs
The reduction in the upper limit of pH for SCC with reduced carbonate concentration was at first thought to be a crack chemistry effect (2).. Now we recognize that this is
species pollinating specialist plants may cause a high mean expected PD loss per lost
Our spatial patch blending technique uses all the patches from which a point p ∈ Ω could have been reconstructed during the inpainting process if the filling order had been
Experiments conducted on several standard natural images illustrate the effectiveness of our method when compared to some other state-of-the-art denoising methods like classical
This experiment deter- mined (1) how the local and neighboring densities of conspecifics affect the movement rates of individuals; (2) how the local and neighboring sex ratios
On pourrait donc s’attendre à ce que les espèces rares, dont les populations sont en général de petite taille, évoluent vers un régime de reproduction autogame.. Or de
Lately, there is an increasing interest on the use of goal-oriented error estimates which help to measure and control the local error on a linear or
Patch construction is a key task in software development. In par- ticular, it is central to the repair process when developers must engineer change operations for fixing the buggy