Fast brain decoding with random sampling and random projections
Texte intégral
Figure

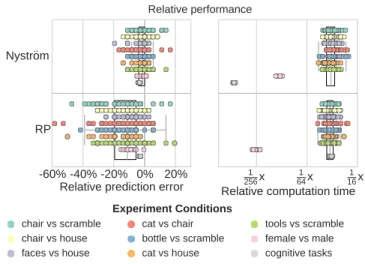
Documents relatifs
We show practical mo- tivation for the use of this approach, detail the link that this random projections method share with RKHS and Gaussian objects theory and prove, both
The fact that fewer large-bodied species went extinct in northern Eurasia is explained by the relatively high reproductive rates of mammal groups there; extinctions cut deeper into
By the same argument we can show that if we map from IR d to IR D using the network obtained by applying the CHR on the data and the quantizers in the low dimensional space,
Several approaches to cluster functional connectivity have been proposed, using various techniques: community detection [15] to discriminate FC characterizing different states of
Figure 10: F reduction for all sparsity configurations using SN-Tucker.. Figure 14: patient 1) : (top) activation profile of HOsSVD (variable V of algorithm 2 (bottom) Cluster of
We consider least-squares regression using a randomly generated subspace G P ⊂ F of finite dimen- sion P, where F is a function space of infinite dimension, e.g.. G P is defined as
However, such a characterization was missing for spectral embedding algorithms such as metric Multi-Dimensional Scaling (MDS) (Torgerson, 1952; Cox and Cox, 1994), spec- tral
The returned object is a list containing the resulting segmentation of variables (segmentation element), a list with matrices of factors for each cluster, mBIC for the chosen