Atomate it! End-user context-sensitive automation using heterogeneous information sources on the web
Texte intégral
Figure


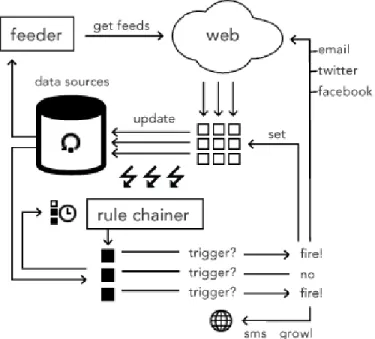
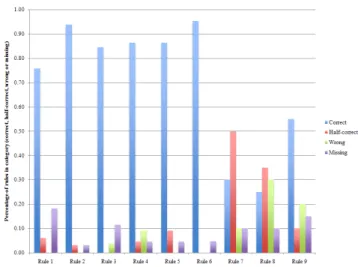
Documents relatifs
In this work, a collaborative tracking framework based on the smartphone’s Wi‐Fi and Bluetooth scanning data is introduced. The Wi‐Fi data is used
In order to better understand user behavior in a multi-intent environment we propose to exploit intent and layout information in a click model so as to improve its performance..
On real data, the cautious rule often yields the best classification results, although we showed that Dempster’s rule, or some rule in- termediate between Dempster’s rule and
The proposed approach uses interval arithmetic to build a graph which has exactly the same number of connected components than S.. Examples illustrate the principle of
Applications for Windows and Android operating systems Two environment options were presented for the users in the experiment: Windows .msi or Android .apk installer files of
What these similar technologies lack is the personal feedback loop be- tween the end user and the technology, where the personal assistant adapts to the end user and the
Concretely, a SimplePARQL query is transformed into N valid SPARQL queries that extend the initial query in order to reach the IRIs or literals in the RDF bases cor- responding
In this paper, we propose a fashion item recommendation method that learns the way the fashion items are matched from a large ensemble database.. We empirically show that the