Sequential Monte Carlo smoothing with application to parameter estimation in non-linear state space models
Texte intégral
Figure
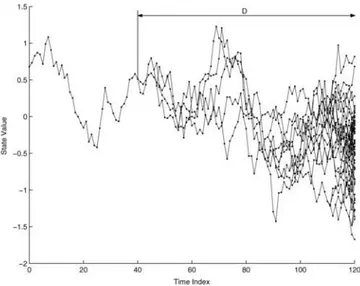
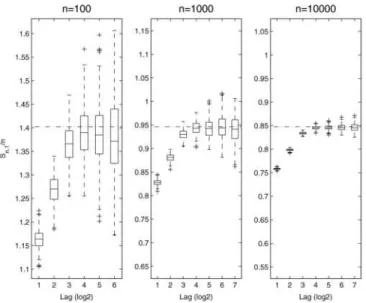


Documents relatifs
A methodological caveat is in order here. As ex- plained in §2.1, the acoustic model takes context into account. It is theoretically possible that the neural network learnt
Nous venons de la rencontrer consisté en l'absence de tout nom et c'est évidement l'anonymat (ex.. ﻈﻨﻤﻟﺍ ﻦﻣ ﺔﻴﺟﺭﺎﺨﻟﺍ ﺕﺎﺒﺘﻌﻟﺍ ﺓﺮﻫﺎﻇ ﻲﺛﺍﺪﺤﻟﺍ ﺭﻮ ﺔﺑﺎﺘﻛ ﻑﺪﻫ ﻥﺇ
Many examples in the literature show that the EI algorithm is particularly interesting for dealing with the optimization of functions which are expensive to evaluate, as is often
• By using sensitivity information and multi-level methods with polynomial chaos expansion we demonstrate that computational workload can be reduced by one order of magnitude
To determine the proper number of particles experimentally, we plotted the average accuracy over 30 experiments with varying the number of particles and problem difficulty in
We describe a quasi-Monte Carlo method for the simulation of discrete time Markov chains with continuous multi-dimensional state space.. The method simulates copies of the chain
Key-words: Hidden Markov models, parameter estimation, particle filter, convolution kernels, conditional least squares estimate, maximum likelihood estimate.. (R´esum´e
Key-words: Hidden Markov models, parameter estimation, particle filter, convolution kernels, conditional least squares estimate, maximum likelihood estimate9. (Résumé