A universal route to pattern formation in multicellular systems
Texte intégral
Figure
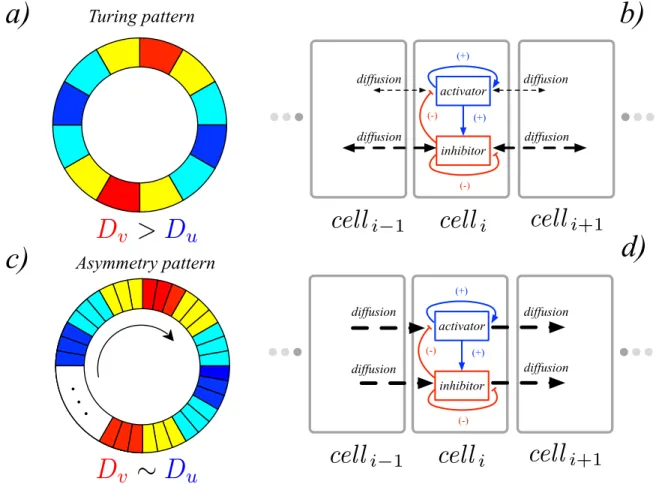

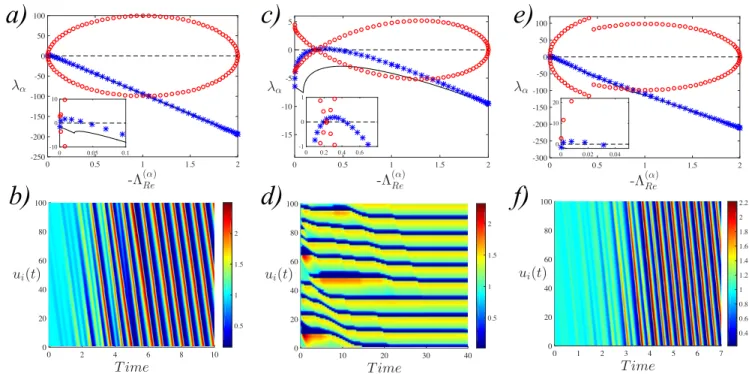

Documents relatifs
These equations govern the evolution of slightly compressible uids when thermal phenomena are taken into account. The Boussinesq hypothesis
Conclusion Most respondents perceived the ratio of specialists to generalists as roughly even and believed the reasons for this balance included increased attention from
But for finite depth foliations (which have two sided branching), there are many examples where it is impossible to make the pseudo-Anosov flow transverse to the foliation [Mo5] and
Let X be a n-dimensional compact Alexandrov space satisfying the Perel’man conjecture. Let X be a compact n-dimensional Alexan- drov space of curvature bounded from below by
In disk storage operations, used to store and control disk sector address.. Retains address of low-order augend digit during addition for
The existence of this solution is shown in Section 5, where Dirichlet solutions to the δ-Ricci-DeTurck flow with given parabolic boundary values C 0 close to δ are constructed..
57.7 and 57.17 : “The following collective rights of indigenous communes, communities, peoples, and nations are recognized and guaranteed, in accordance with the Constitution
In a system like Emerald that does provide testable object identity, Gaggles can be used to hide it, by creating multiple object identifiers for an object, as described in the