Unsupervised amplitude and texture classification of SAR images with multinomial latent model
Texte intégral
Figure


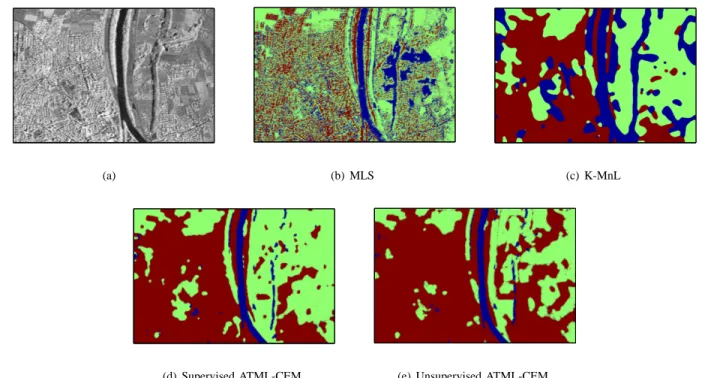

Documents relatifs
Using simple first-order Arrhenius kinetics
In accordance with the absence of any detectable IFN-α production, the blocking of type I IFN signaling had no effect on TRAIL and CXCL10 expression induced by
Antenna tips are now electropolished (Figure 6), and the number and size of particles is checked at every step of the preparation in the clean room, as it is for every
heterodyne self-oscillator approach [7] capable of simultaneously tracking two modes of resonance of a NEMS without degrading the resonator mass resolution and sensitivity. Section
We propose an original approach coupling ab initio calculations and linear elasticity theory to obtain the properties of the isolated point defect for reduced supercell sizes..
The cell esds are taken into account individually in the estimation of esds in distances, angles and torsion angles; correlations between esds in cell parameters are only used
REPUBLIQUE ALGERIENNE DEMOCRATIQUE ET POPULAIRE MINISTERE DE L’ENSEIGNEMENT SUPERIEUR ET DE LA
Cette opération se fait avec l’opérateur Insérer arc-plan entre Extension (Proc[M]) et < [Élément X] ; Etendre, par DECOMPOSITION GLOBALE (Greffe Additionnelle), [Élément