Benchmarking MRI Reconstruction Neural Networks on Large Public Datasets
Texte intégral
Figure
![Figure 1: Illustration of the U-net from [27]. In our case the output is not a segmentation map but a reconstructed image of the same size (we perform zero-padding to prevent decreasing sizes in convolutions).](https://thumb-eu.123doks.com/thumbv2/123doknet/13461057.411664/7.918.213.703.198.534/figure-illustration-segmentation-reconstructed-perform-padding-decreasing-convolutions.webp)
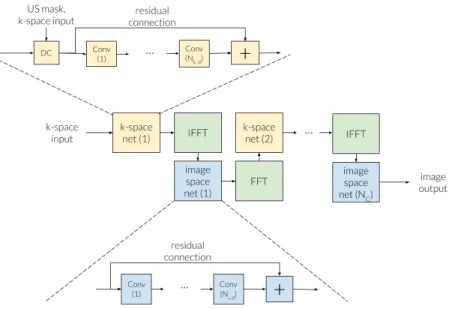
![Figure 4: Illustration of the KIKI-net from [9]. The KCNN and ICNN are convo- convo-lutional neural networks composed of a number of convoconvo-lutional blocks varying between 5 and 25 (we implemented 25 blocks for both KCNN and ICNN), each followed by a R](https://thumb-eu.123doks.com/thumbv2/123doknet/13461057.411664/9.918.212.707.455.591/illustration-lutional-networks-composed-convoconvo-lutional-implemented-followed.webp)
![Figure 5: Illustration of the PD-net from [8]. Here T denotes the measurement operator, which in our case is the under-sampled Fourier transform, T ∗ its adjoint, g is the measurements, which in our case are the undersampled k-space measurements, and f 0](https://thumb-eu.123doks.com/thumbv2/123doknet/13461057.411664/10.918.213.716.191.371/illustration-measurement-operator-fourier-transform-measurements-undersampled-measurements.webp)
Documents relatifs
L’archive ouverte pluridisciplinaire HAL, est destinée au dépôt et à la diffusion de documents scientifiques de niveau recherche, publiés ou non, émanant des
The question is about the relevancy of using video on which temporal information can be used to help human annotation to train purely image semantic segmentation pipeline
In Table 2, we report the reconstruction PSNRs obtained using the proposed network, and for a network with the same architecture where the mapping is learned (‘Free Layer’)
Our simulation shows than the proposed recur- rent network improves the reconstruction quality compared to static approaches that reconstruct the video frames inde- pendently..
Table 1: Average peak signal-to-noise ratio (PSNR) and average structural similarity (SSIM) over the UCF-101 test dataset for the three different methods, as the least-squares
The results obtained in the reliability prediction study of the benchmark data show that MLMVN out- performs other machine learning algorithms in terms of prediction precision and
In practice the modulation amplitude is always smaller than this length because one great advantage of a broadband source is the possibility of using objectives with a depth of
Figure 8.6 shows converter efficiency versus modulation frequency when the converter is driven with duty cycles of 20, 50, and 80%, for an input voltage of 14.4 V, and a fixed