Generating Adversarial Examples for Topic-dependent Argument Classification
Texte intégral
Figure
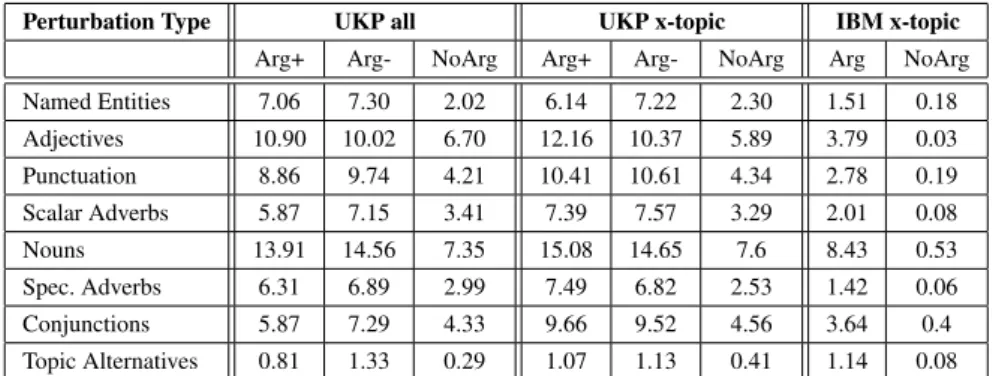

Documents relatifs
4 Next, in the first chapter, we respond directly to the devil’s advocate, by experimentally testing whether a constrained set of evidence (i.e., a handful of known words and
Classification models (“machine learners” or “learners”) were developed using machine learning techniques to predict mortality at discharge from an intensive care unit (ICU)
In this paper we investigate the question of whether modern, highly-optimized de- scription logic reasoners are robust over Web input. The general intuition of a robust system is
In this paper we investigate the question of whether modern, highly-optimized de- scription logic reasoners are robust over Web input. The general intuition of a robust system is
Abstract We present a new data set of sulfur dioxide (SO 2 ) vertical columns from observations of the Ozone Monitoring Instrument (OMI)/AURA instrument between 2004 and 2013..
The methodology is illustrated on the line following problem of a sailboat and then validated on an actual experiment where an actual sailboat robot, named Vaimos, sails
A first example of this approach could be the extension of an organization’s knowledge base to documents related to its territorial and social context: in this way the
This conjecture, which is similar in spirit to the Hodge conjecture, is one of the central conjectures about algebraic independence and transcendental numbers, and is related to many