Bayesian Pursuit Algorithms
Texte intégral
Figure


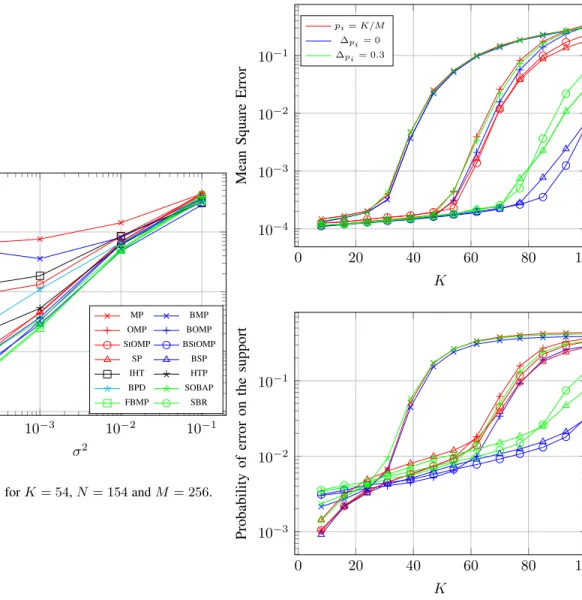
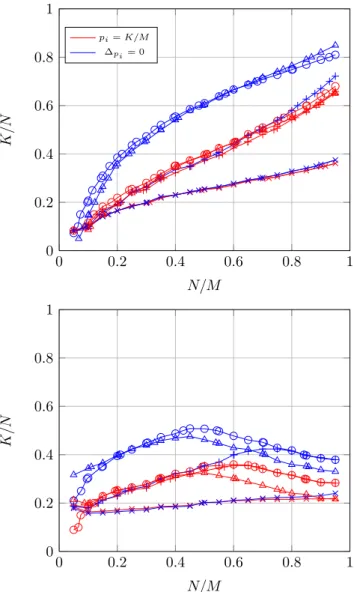
Documents relatifs
A general overview of analytical and iterative methods of reconstruction in computed tomography (CT) is presented in this paper, with a special focus on
∇η B (θ) into a GP term and a deterministic term, 2) using other types of kernel functions such as sequence kernels, 3) combining our approach with MDP model estimation to al-
More work is required to close the gap and suggest a theoretical oracle- like denoising guarantee for the stagewise version of these
We obtain a Central Limit Theorem for a general class of addi- tive parameters (costs, observables) associated to three standard Euclidean algorithms, with optimal speed
Since the training set shows very different shapes for the six categories, a single template model might not be able to capture this large variability.. In order to have a little
These results show that randomized comparison-based algorithms are not so bad, in spite of their limitations in terms of convergence rate: (i) they are optimal in the robust
These results for the single signal approximation case suggest that in the simultaneous sparse approximation problem, reweighted M-Basis Pursuit would lead to sparser solutions than
Given their similarity, we explore in this paper both greedy and working set strategies to solve respectively the problems penalized by the Mumford-Shah penalty for piecewise