Spectral inference methods on sparse graphs : theory and applications
Texte intégral
Figure
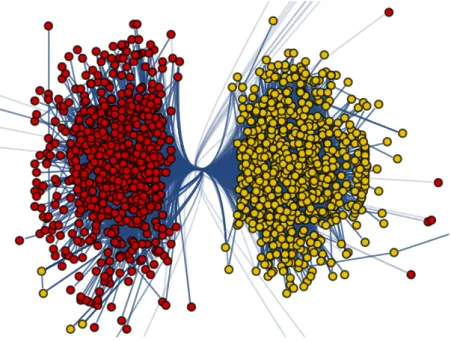
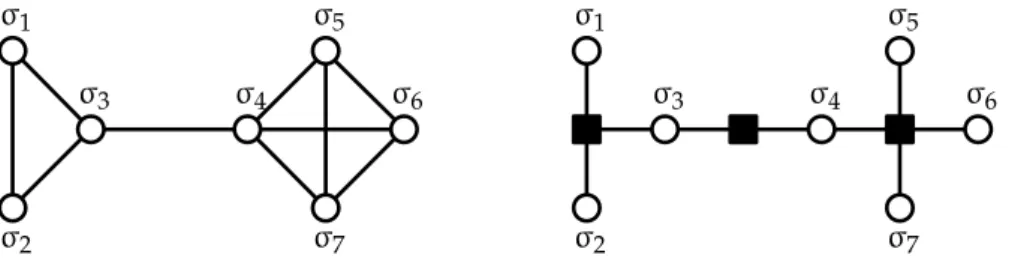


Documents relatifs
Before extending it to unbounded normal operator we develop here the Spec- tral Theorem for bounded normal operators, under its two main forms: the functional calculus form and
We will consider the problem of finding suitable vertex orders for graphs representing instances of the distance geometry problem [13], as well as one variant of the
(French) [Some regular elements of Weyl groups and the associated Deligne-Lusztig varieties] Finite reductive groups (Luminy, 1994), 73–139, Progr.. Lusztig, Representations
They showed that the problem is NP -complete in the strong sense even if each product appears in at most three shops and each shop sells exactly three products, as well as in the
The resulting model has similarities to hidden Markov models, but supports recurrent networks processing style and allows to exploit the supervised learning paradigm while using
Our algorithm is of particular interest to compute the Ate-style pairings [3, 17] on elliptic curves with small embedding degrees k, and in situations where denominator elimina-
Using Matlab, we first generate random graphs with an exact group structure. We choose different number of blocks and different sizes for the blocks. Then, we add a noise on
Extensive experimentations have been conducted for comparing our algorithm on various families of weighted graphs against the most efficient practical algorithms for the SSSP