Malware Detection in PDF Files Using Machine Learning
Texte intégral
Figure

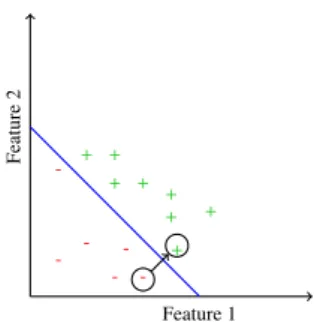
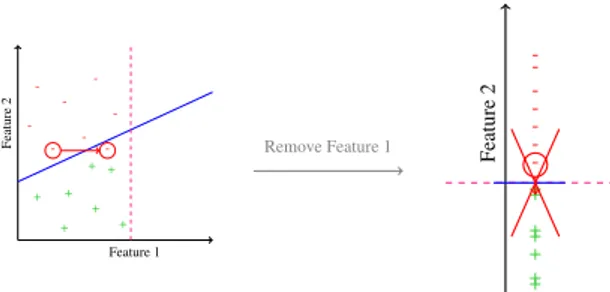
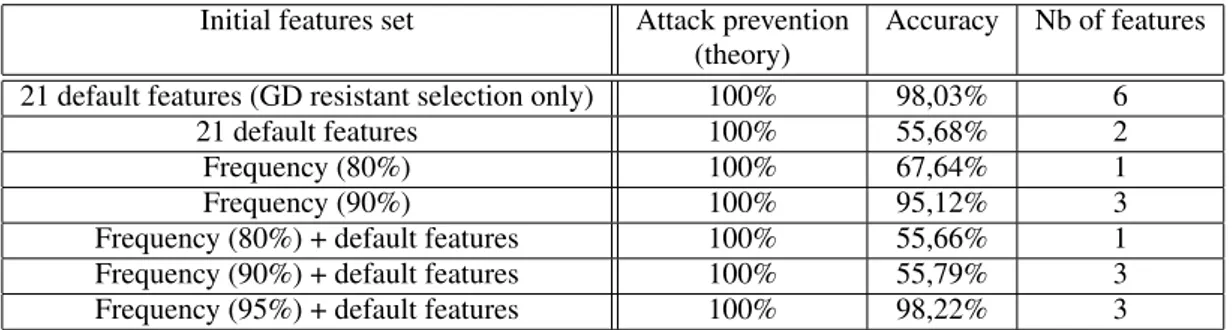
Documents relatifs
Decisions of the eighth session of the Committee (2013) Decisions 8.COM Decisions of the seventh session of the Committee (2012) Decisions 7.COM Decisions of the sixth session of
Por favor, seleccione los marcos azul para acceder al
Please click on the different sections for access to the
Range bound errors are always detected, unless runtime checks are disabled.. set
[14] Konstantin Pogorelov, Michael Riegler, Pål Halvorsen, Carsten Gri- wodz, Thomas de Lange, Kristin Ranheim Randel, Sigrun Eskeland, Dang Nguyen, Duc Tien, Olga Ostroukhova,
Since we use this approach only with malicious files, Adversarial Occlusion is an especially challenging test for our machine learning based methods: they have seen these byte
The key idea is that we collect meta- data about the attributes in the database (e.g., attribute data type), meta-data about the feature generative functions (e.g., id of the
The convexity hull defects are also used for anthropometrical features extraction, then the support vector machine (SVM) will be applied for gender classification.. Cluster