Robustness of the linear mixed model to misspecied error distribution: Robustness of the linear mixed model
Texte intégral
Figure
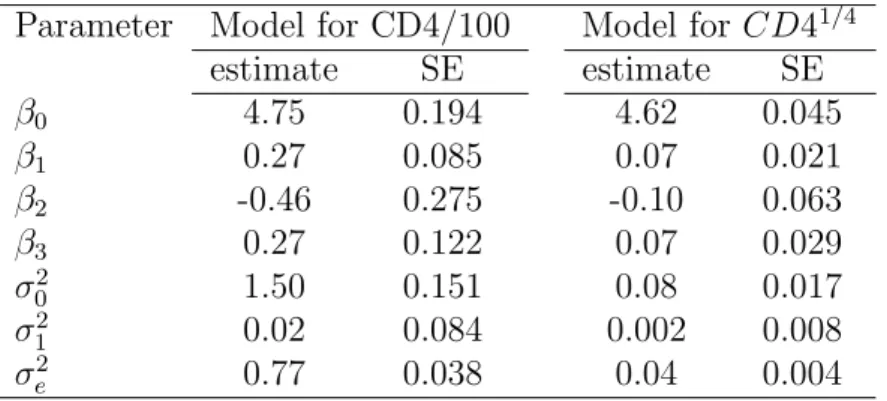


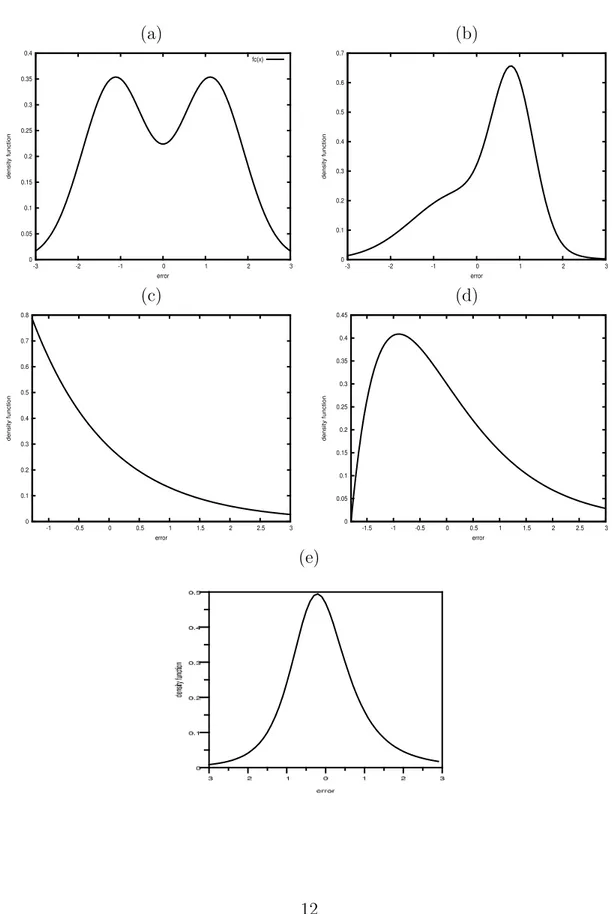
Documents relatifs
It is worth noting that the optimality of p = 1/n apparently was never proven rigorously before, not even for the case of OneMax 2 , where tight upper and lower bounds on the
In a second step, we used the typology in order to build a model of the spatial structure, that can simulate realistic virtuals stands from data at the
We used the cartography of 25 1ha plots in Orléans forest (1) to analyse the spatial structure (2) and to build a typology of Sessile oak-Scots pine mixed stands. This
Summary - The analysis of threshold models with fixed and random effects and associated variance components is discussed from the perspective of generalized linear mixed
versions of the Gibbs sampler for a univariate normal mixed linear model assuming prior independence of the variance components. The Gibbs sampler algorithm generates
In this paper, sufficient conditions for the robust stabilization of linear and non-linear fractional- order systems with non-linear uncertainty parameters with fractional
First, the noise density was systematically assumed known and different estimators have been proposed: kernel estimators (e.g. Ste- fanski and Carroll [1990], Fan [1991]),
In both models with ME (with and without misclassification), when the variance of one measurement error increases, the bias of the naive estimator of the coefficient parameter of