Extending Approximate Bayesian Computation with Supervised Machine Learning to infer demographic history from genetic polymorphisms using DIYABC Random Forest
Texte intégral
Figure
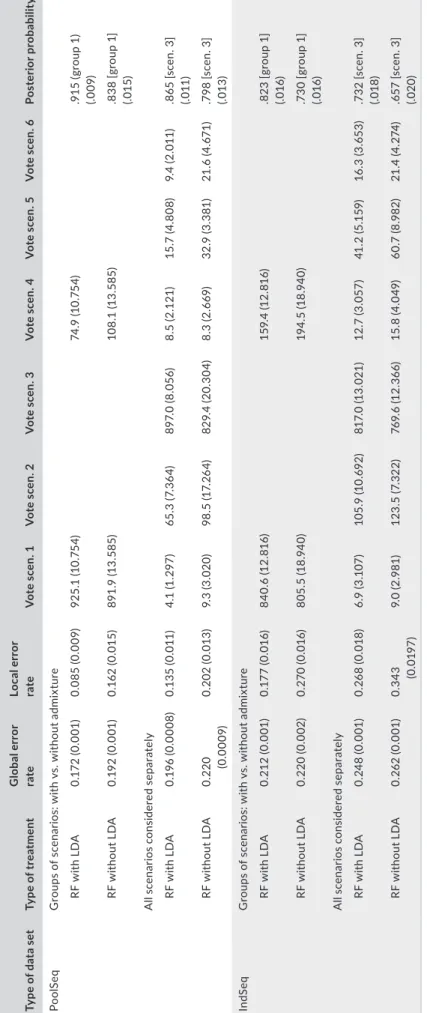
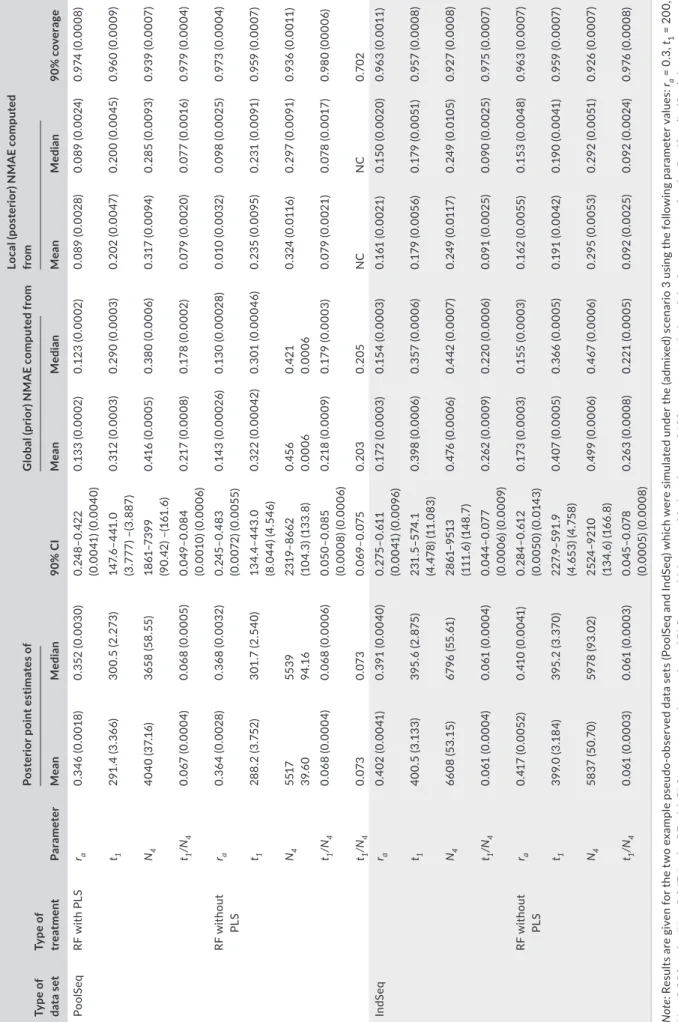
Documents relatifs
This whole process is twofold. The exploitation of syntactic paths as extraction patterns allows the discovery of distant NE pairs which are difficult to identify in the
megalosiphon inhibitor of microbial serine proteinase gene (NmIMSP) rapidly induced during the interaction. Silencing of NmIMSP gene was found to compromise the resistance of
Four major findings are obtained: (i) The WTP for urban park proximity is globally non-significant compared with that of forest areas; (ii) When forests are recreational
As shown in the above algorithm, the main difference between Forest-RI and Forest-RK lies in that K is randomly chosen for each node of the tree leading therefore to more diverse
Finally, on online random forests we perform incremental learning feature-by-feature (i.e.: first we train the random forest on the first feature, then we add the second feature
combining the view-specific dissimilarities with a dynamic combination, for which the views are solicited differently from one instance to predict to another; this dynamic
In the work [ 44 ], the authors identify Radiomics as a challenge in machine learning for the three following reasons: (i) small sample size: due to the difficulty in data sharing,
The model choice proce- dure is efficient if the TPR is close to 1 for both following cases: when the data sets are simulated under a constant population size (avoiding overfitting