Hybrid Brain-Computer Interfaces: Improving Mental Task Classification Performance through Fusion of Neurophysiological Modalities.
Texte intégral
Figure
![Figure 2.1: Structure of a standard hBCI. This functional model is inspired by [26] and [24].](https://thumb-eu.123doks.com/thumbv2/123doknet/5390512.125350/59.918.135.772.122.426/figure-structure-standard-hbci-functional-model-inspired.webp)
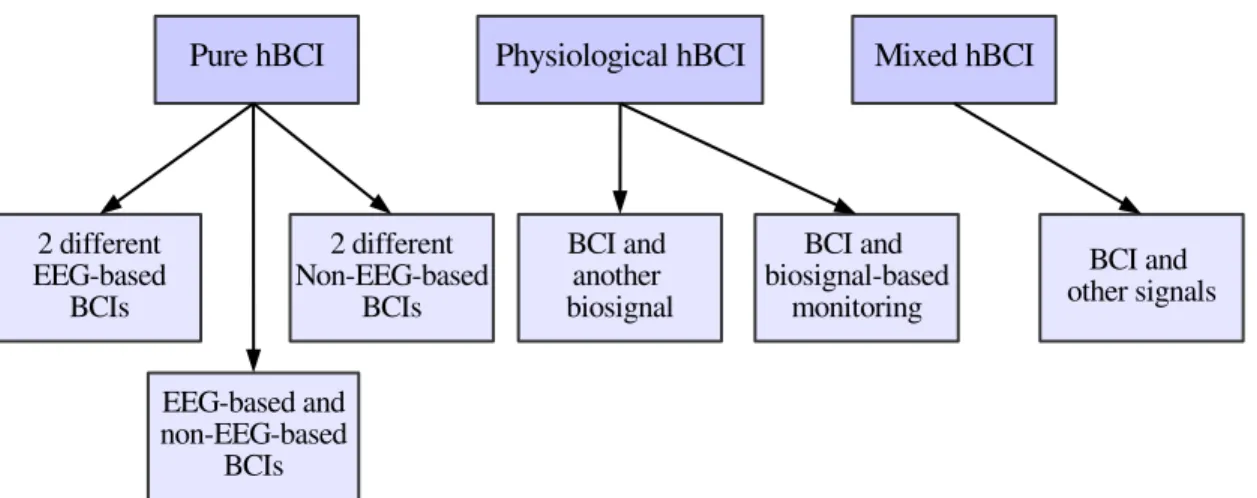
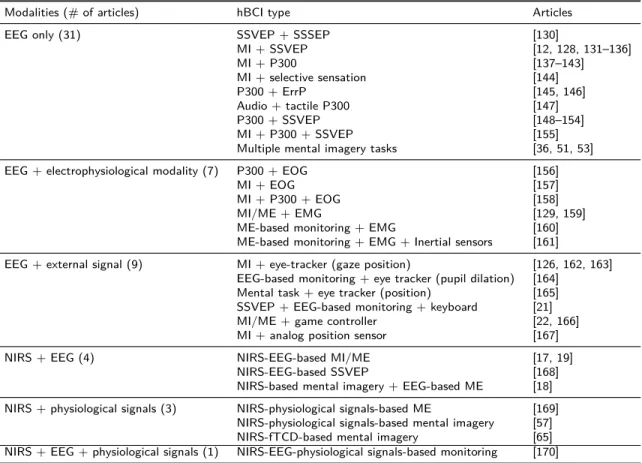
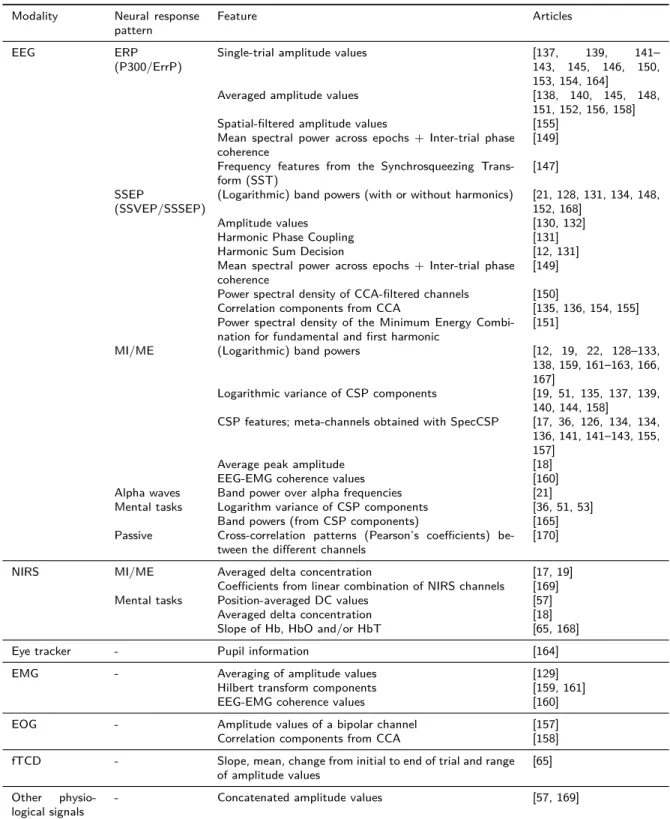
Outline
Documents relatifs
In this paper, we propose to overcome these limitations by (1) con- sidering users’ mean performances across 6 sessions to get a better idea of their longer-term MI-BCI control
In this chapter, we have provided a tutorial and overview of EEG signal processing tools for users’ mental state recognition. We have presented the importance of the feature
They are divided into five different categories: linear classifiers, neural networks, nonlinear bayesian classifiers, nearest neighbor classifiers and combinations of classifiers..
We could make the hypothesis that the adaptation of haptic assistance based on mental workload index would help the user and would result in an increase in task performance..
For instance, a typical BCI training session provides a uni-modal (visual) and corrective feedback (indicating whether the learner performed the task correctly) (see Figure 1),
Several approximation methods for Gaussian process classification are employed in this paper and the experimental results suggest that across all the data sets employed, all GP
Several classification al- gorithms have been used to design BCI, such as linear classifiers, Support Vector Machine (SVM) or neural networks [9].. Surprisingly, fuzzy classifiers
The method enabled us to select the labels that were best classified by the algorithm and therefore that were likely to give the best results in asynchronous BCI, assuming that the