Contributions to Monte Carlo Search
Texte intégral
Figure

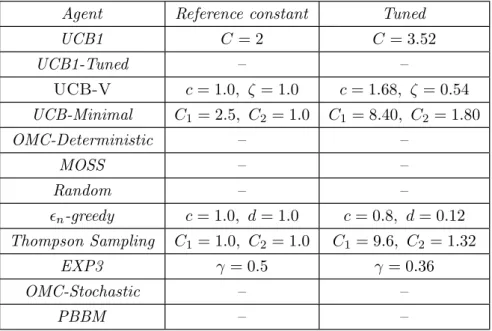


Documents relatifs
The case with different player roles assumes that one of the players asks questions in order to identify a secret pattern and the other one answers them.. The purpose of the
• In the second chapter ( Existence of an optimal strict control and optimality conditions for linear FBSDEs of mean-field type): In this chapter, we prove the existence of a
This results should leads us to dene a less RMS than 1 nm for the specication but we should take care with this conclusion because the PSD used for the SESO maps are
The existence of Hapalemur in a region approximately halfway between the popu- lations in the far north-west and in the west would further suggest that Hapalemur may once have had
Monte Carlo Tree Search (MCTS) [5] is a recent algorithm for taking decisions in a discrete, observable, uncertain environment with finite horizon that can be described as
Copyright and moral rights for the publications made accessible in the public portal are retained by the authors and/or other copyright owners and it is a condition of
Copyright and moral rights for the publications made accessible in the public portal are retained by the authors and/or other copyright owners and it is a condition of
– As a by product of this work, we’ll see the interesting property that some heavily tuned MCTS implementations are better than UCT in the sense that they do not visit the complete