Detecting Illicit Entities in Bitcoin using Supervised Learning of Ensemble Decision Trees
Texte intégral
Figure


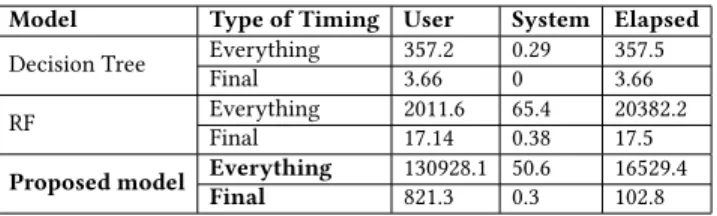
Documents relatifs
Diagram of the proposed approach has 6 steps: (1) The voice examination begins; (2) Voice signal acquisitions equipment; (3) Preprocessing audio signals; (4) Feature extraction
Furthermore, the graph-based analysis of Bitcoin transactions can help discern suspicious transaction behaviors and currency flows involving Bitcoin users.. Future work will focus
In this article, we show how the Bitcoin transac- tion network can be studied using complex networks analysis techniques, and in particular how community detection can be
In IBD, machine learning has been used to classify IBD paediatric patients using endoscopic and histological data 15 , to distinguish UC colonic samples from control and
Features learned by a convo- lutional neural network (CNN), bottleneck features from existing models and manually extracted features from the spectrograms comprised the three
Using greedy algorithms to learn them can pose several disadvantages: it is difficult to limit the size of the decision trees while maintaining a good classification accuracy, and it
This shows that the quantity of the aggressive tweets (the class of interest) was the half of the non- aggressive tweets. This is usual in many text classification problems.
This paper presents a machine learning approach for detecting structural stiffness changes of civil engineering structures considered as dynamical systems, using only an