The Tamed Unadjusted Langevin Algorithm
Texte intégral
Figure


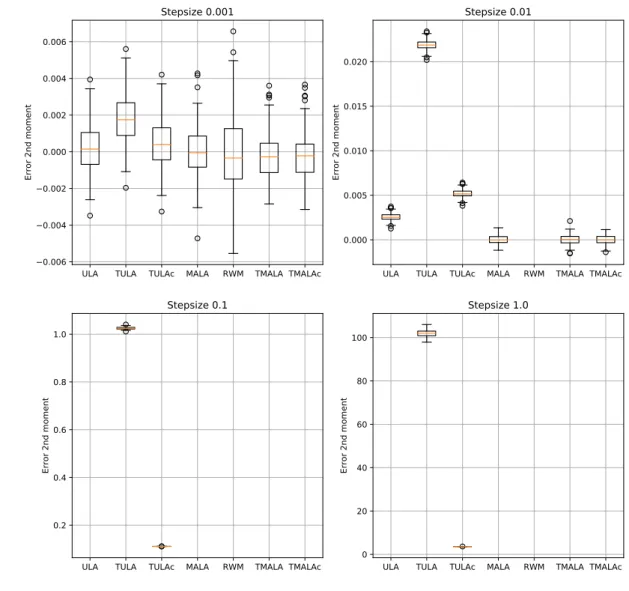

Documents relatifs
Among quantum Langevin equations describing the unitary time evolution of a quantum system in contact with a quantum bath, we completely characterize those equations which are
We justify the efficiency of using the explicit tamed Euler scheme to approximate the invariant distribution, since the computational cost does not suffer from the at most
This method is based on the Langevin Monte Carlo (LMC) algorithm proposed in [19, 20]. Standard versions of LMC require to compute the gradient of the log-posterior at the current
The dependency on the dimension and the precision is same compared to [1, Proposition 2] (up to logarithmic terms), but this result only holds if the initial distribution is a
L’accès à ce site Web et l’utilisation de son contenu sont assujettis aux conditions présentées dans le site LISEZ CES CONDITIONS ATTENTIVEMENT AVANT D’UTILISER CE SITE WEB.
More precisely, the objective of this paper is to control the distance of the standard Euler scheme with decreasing step (usually called Unajusted Langevin Algorithm in the
However, the pattern of interconnected microcolonies cannot be obtained with these usual mechanisms: immotile bacteria form isolated microcolonies and constantly
We establish for the first time a non-asymptotic upper-bound on the sampling error of the resulting Hessian Riemannian Langevin Monte Carlo algorithm.. This bound is measured