Learning and Recognizing Human Action from Skeleton Movement with Deep Residual Neural Networks
Texte intégral
Figure
![Figure 1. The position of the joints extracted by Kinect [21].](https://thumb-eu.123doks.com/thumbv2/123doknet/3069759.86680/3.892.476.807.123.251/figure-position-joints-extracted-kinect.webp)
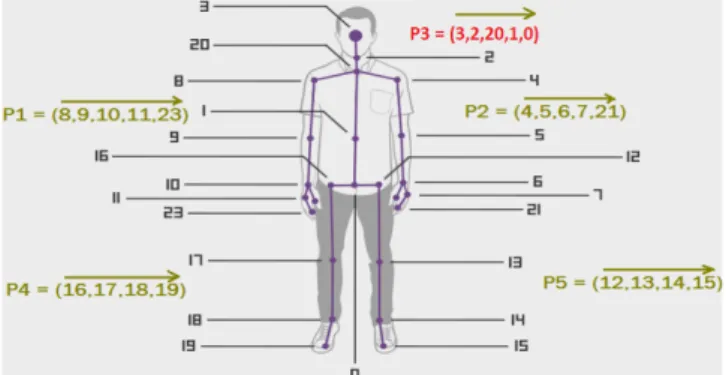
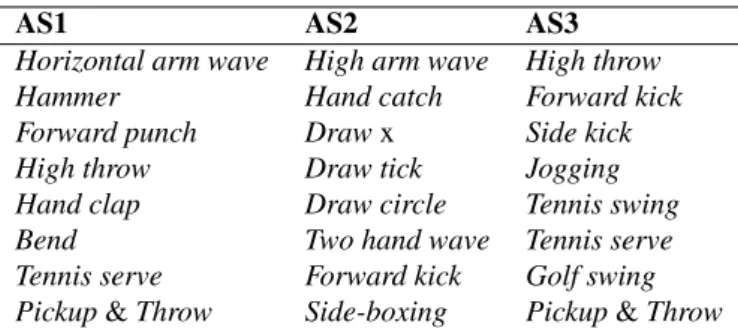
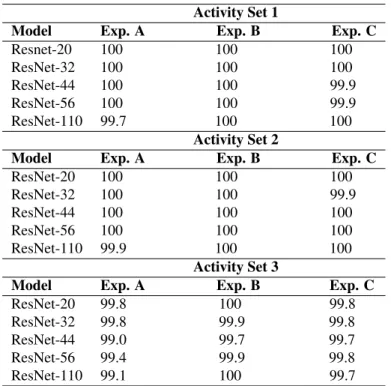
Documents relatifs
In Table 1, we report our single-image autoencoder-based results on this dataset along with those of the following state- of-the-art single image-based methods: KDE regression from
Wang, “Hierarchical recurrent neural network for skeleton based action recognition,” in IEEE conference on computer vision and pattern recognition (CVPR), 2015, pp. Schmid,
Our proposed model, stochastic residual network (S-ResNet), employs stochastic shortcut connections from the input to the hidden layers for essentially improving the
Abstract— In this paper, we propose a new hand gesture recognition method based on skeletal data by learning SPD matrices with neural networks.. We model the hand skeleton as a
All the feature vectors are then efficiently com- bined by a metric learning method inspired by the LMNN algorithm, which is used to extract the most discriminant information
The proposed method for action recognition can be seen as composed by two parts: one as pose-based recognition, which uses a sequence of body joints coordinates to predict the
Key Words: human activity recognition, skeleton data, deep learning, residual neural net- work, cell internal relationship, spatio-temporal information.. ∗ E-mail