Variational channel estimation with tempering: an artificial intelligence algorithm for wireless intelligent networks
Texte intégral
Figure

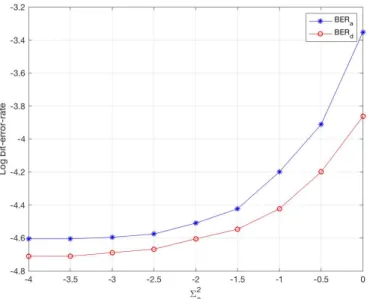
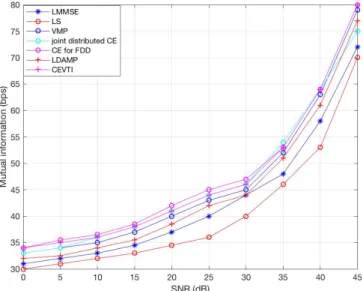
Documents relatifs
Note that most classical massive MIMO channel estimation methods assume a similar physical model, but discretize a priori the DoDs and DoAs, so that the problem fits the framework
Using the maximum entropy principle to exploit prior system information at the receiver, we successively derive channel estimates in situations when (i) the channel delay spread
We then perform an LMMSE estimation of the sum of the physical and artificial channel using the filter covariance matrix and the channel estimation is obtained by subtracting
(11) In summary, the channel depends on the 7P physical param- eters φ given in (3), but the considered model depends on only 7p parameters whose optimal values make up the
Association Optimization in Wi-Fi Networks based on the Channel Busy Time Estimation.. Mohammed Amer, Anthony Busson,
The first step involves a large SVD in order to obtain a base for the noise subspace of the received signal, and the second consists in applying either a small SVD [8] or
In this paper, we propose an adaptive channel estimation based on deep learning that assumes the signal-to-noise power ratio (SNR) knowledge at the receiver, and we show that
The proposed blind channel estimation method is based on the second order statistics of the received signal, in addition, the algorithm exploits knowledge of the desired spreading