Acceleration Strategies of Markov Chain Monte Carlo for Bayesian Computation
Texte intégral
Figure
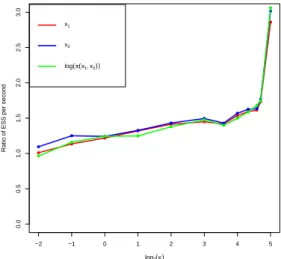
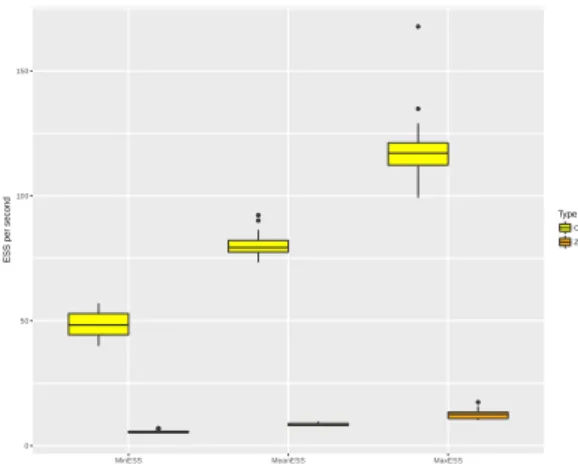


Documents relatifs
Keywords Bayesian statistics · Density dependence · Distance sampling · External covariates · Hierarchical modeling · Line transect · Mark-recapture · Random effects · Reversible
Table 3 presents the results for these cases with an a priori spectral index equal to the real value α = 1.1 (case IIIa), and without any a priori value on any parameter (case IIIb)
Comparison between MLE (CATS) and a Bayesian Monte Carlo Markov Chain method, which estimates simultaneously all parameters and their uncertainties.. Noise in GPS Position Time
– MCMC yields Up component velocity uncertainties which are around [1.7,4.6] times larger than from CATS, and within the millimetre per year range
Key words: Curve Resolution, Factor analysis, Bayesian estimation, non-negativity, statistical independence, sparsity, Gamma distribution, Markov Chain Monte Carlo (MCMC)..
The proposed model is applied to the region of Luxembourg city and the results show the potential of the methodologies for dividing an observed demand, based on the activity
The posterior distribution of the parameters will be estimated by the classical Gibbs sampling algorithm M 0 as described in Section 1 and by the Gibbs sampling algo- rithm with
Probabilities of errors for the single decoder: (solid) Probability of false negative, (dashed) Probability of false positive, [Down] Average number of caught colluders for the